Convolutional Neural Networks used to Detect Landslides in RapidEye Imagery
Landslides pose a major threat to people and property throughout the world, and particularly in landslide-prone regions. Improving our ability to monitor those landslides can improve mitigation, and may ultimately reveal approaches that could predict certain types of landslides in the future. Omid Ghorbanzadeh, from the University of Salzburg, and his team applied convolutional neural networks and other methods to identify landslides in RapidEye data. Working in the Rasuwa district in Nepal, Omid and team tested their ability to detect thousands of landslides based on a variety of image spectral features from RapidEye data, as well as topographic inputs including digital elevation models from ALOS data. The results were encouraging. Across all methods, their model precision ranged from 50 - 82%, while recall ranged from 60 - 93%. The group found that spectral features from RapidEye data were more important than topography for identifying landslides. They conclude that: “the increasing availability of [Very High Resolution] remotely sensed imagery opens many options for landslide mapping and for producing and updating landslide inventories.” The authors caution, however, that landslide mapping remains challenging due to the wide diversity of forms landslides can take. The full paper is available via open access from the journal Remote Sensing. [caption id="attachment_143221" align="aligncenter" width="1321"]
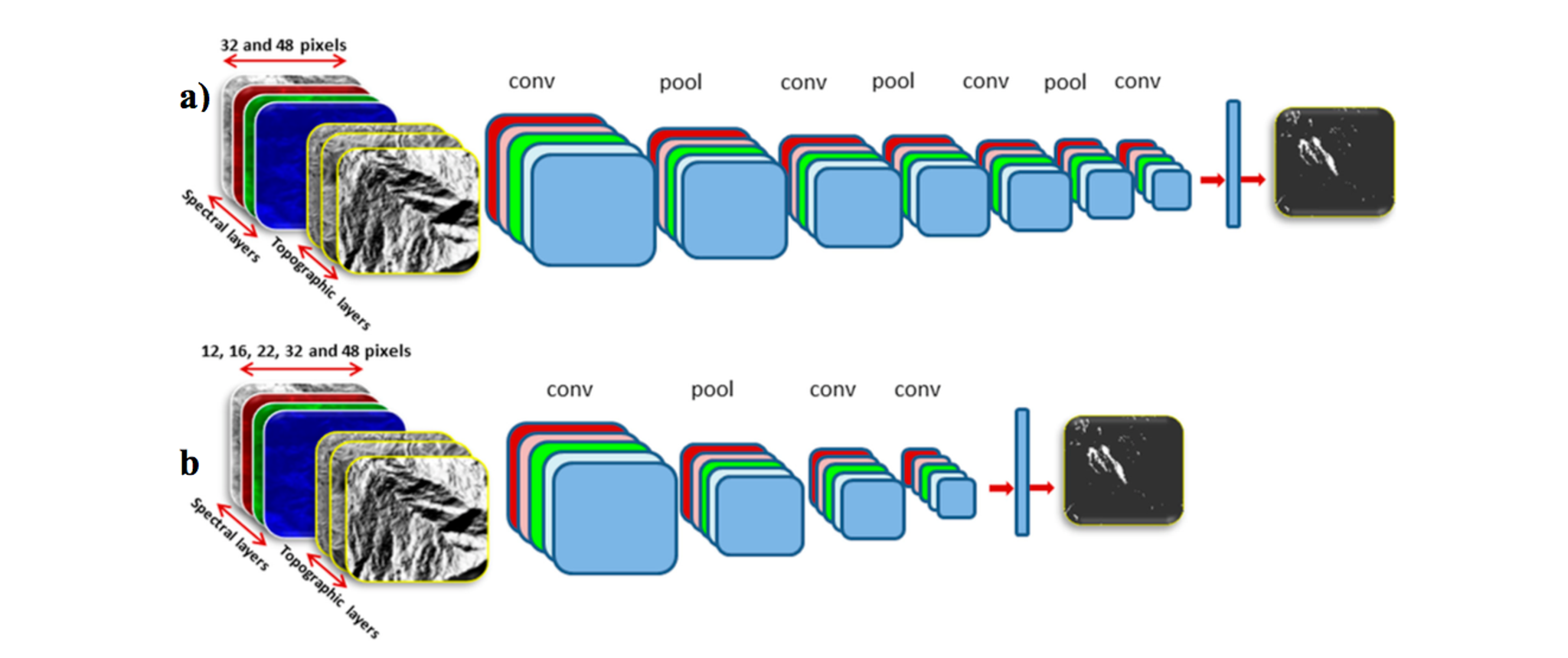
Ready to Get Started
Connect with a member of our Sales team. We'll help you find the right products and pricing for your needs