Forest Biomass Monitoring with the RapidEye Constellation
When working with forest carbon stocks and emissions, it is notable that there is no strictly “true” data. In order to directly measure carbon in forests, one would need to cut the vegetation, dry it, weigh it, chip it up, and push it through a mass spectrometer. This being a self-defeating enterprise, scientists have for decades estimated carbon content of forests in the field using a mix of volumetric measurements (e.g. tree diameters and height), as well as allometric equations: mathematical relationships between tree volume parameters and carbon measurements collected from harvesting and weighing individual trees. With such equations, one can produce field estimated carbon stocks . However, the limits of measuring trees in the field are astronomical. In recent years, airborne LiDAR (light detection and ranging) has allowed scientists to produce similar (but not identical) volumetric measurements of forests from the air. But, LiDAR remains expensive to deploy. If high-resolution satellite imagery, such as Planet’s RapidEye or Dove data, could be used to sense the volumetric structure of forests, dramatic improvements in carbon mapping would be possible. Lorena Hojas Gascón, from Universitat de València, and her team used RapidEye data to support a country wide biomass inventory in Tanzania. Using a RandomForest algorithm, the authors were able to explain 69% of variation in forest biomass throughout the country. The authors conclude that “optical data of finer spatial resolution, in this case 5 m resolution, can be used to obtain biomass estimates at a national level.” The full paper is available via open access from the journal Forests. [caption id="attachment_143198" align="aligncenter" width="918"]
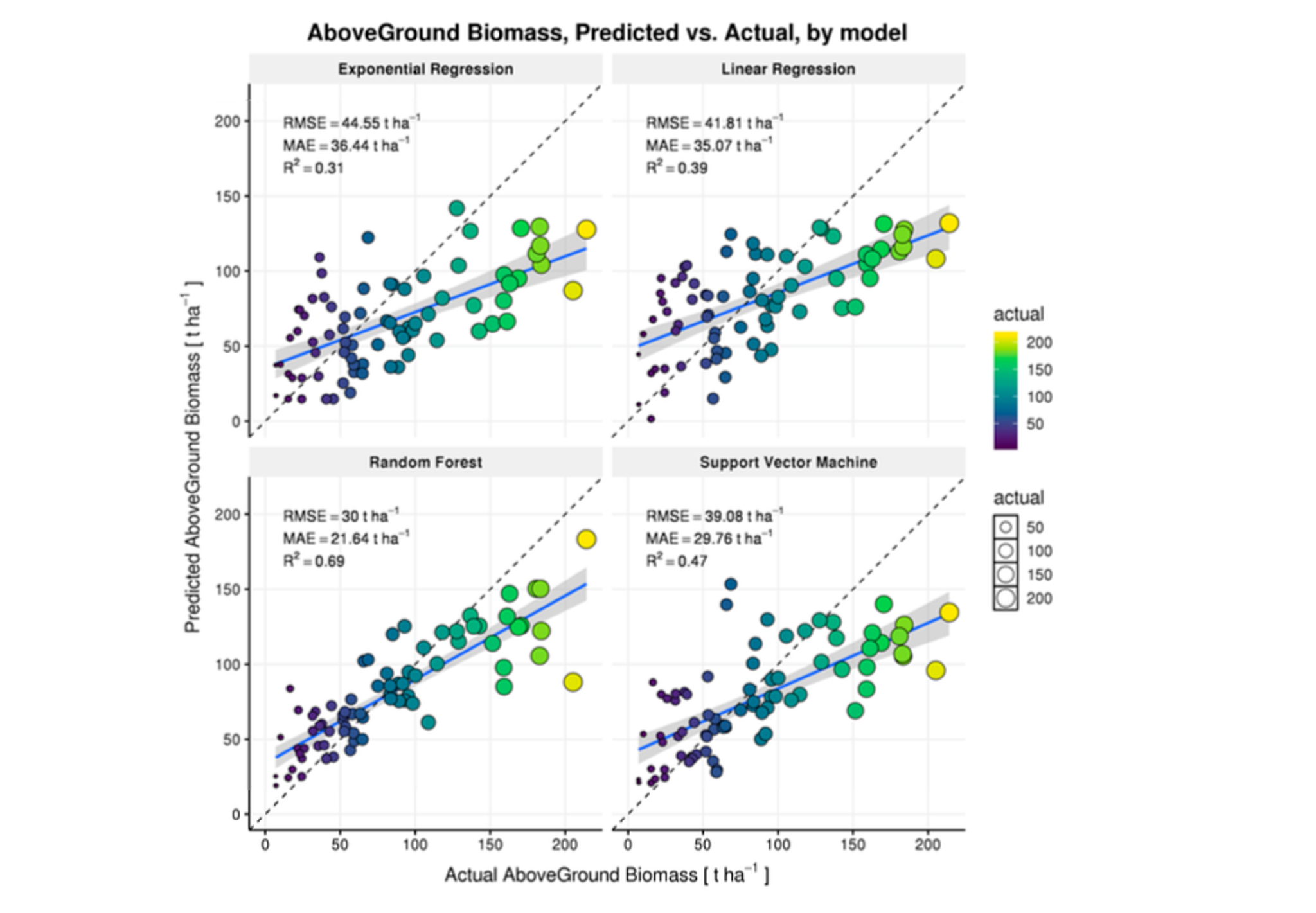
Ready to Get Started
Connect with a member of our Sales team. We'll help you find the right products and pricing for your needs