Planet’s Framework for Analysis Ready Data
Anyone who works closely with geospatial data knows that there is a great deal of labor involved in preparing the data before analysis begins. In fact, much of modern geoprocessing systems are set up to make the data ready for analysis.
The industry understands that geospatial data must become more user-friendly and accessible if it is to be leveraged by broader audiences. Out of this context, the idea of “analysis ready data” (ARD) emerged. An easy way to think of ARD is deep stacks of data prepared for someone to analyze with all of the pre-processing work already completed.
At Planet, we are doing a lot of thinking about how to make it even easier for our user community to bypass pre-processing steps and dive into the interesting stuff – analysis. While much of this work is evolving, we can say today that there are a number of key ingredients of ARD that matter at Planet.
Radiometry – Calibration and Atmospheric Correction
We dedicate a lot of resources to ensuring the radiometric integrity and quality of our imagery. There are a number of unique challenges that Planet must address given the number of satellites in the constellations we are managing. The biggest one is maintaining consistency between sensors and over time. We keep radiometry consistent by cross-calibrating Doves, RapidEye, and SkySats using the same ground calibration targets, and having a consistent process for converting our data to surface reflectance. Using surface reflectance allows users to apply the same algorithms to different data sources and get consistent results that aren’t impacted by the effects of the atmosphere over time. Surface reflectance assets are currently available for PlanetScope imagery, and will soon be available for RapidEye.

A true color comparison of the surface reflectance product (top) and a top of atmosphere reflectance image (bottom) in adjacent scenes captured by the same satellite.
Geometry – Orthorectification and Co-registration
Orthorectification is the process of correcting image geometry, such as the removal of distortion caused by view angle and terrain, so that the coordinates of each pixel are projected to an accurate location on Earth. Planet uses a highly accurate, high-resolution ground control point (GCP) data set to achieve positional accuracy of less than 10 meters RMSE globally (usually less than 5 meters observed during our regular testing).
Metadata – Usable Data Masks (UDM)
Planet provides a simple usable data mask (UDM) asset with all imagery items today. This asset includes information on whether a pixel in an image contains usable imagery, cloud, nodata, or “anomalous” pixels (e.g., hot pixels, saturation, etc.). While this allows a user to quickly assess which pixels to trust, it does not yet provide nuanced information about whether a pixel is affected by cloud shadow or haze. We are currently working to improve cloud masking methods that will harmonize our processes across the RapidEye, Skysat, and PlanetScope constellations, improve accuracy of the cloud identification, and add information about cloud shadow and haze. Planet uses the same information internally to select which cloud-free data to include in our surface reflectance basemaps.
Interoperability – Sensor-to-Sensor Normalization
There are a few different types of optical imagery available to users through the Planet platform, including Landsat, Sentinel-2, RapidEye, PlanetScope, and more to come. Between these sensors there are small differences in the relative spectral response of common bands, so if you are trying to compare results of an NDVI when using different data sets, it is helpful to normalize these differences to avoid added uncertainty in the comparison. Planet will soon provide the equivalent of spectral band adjustment factors for all of the electro-optical data sets available in the platform so, for example, the red band of RapidEye looks like the red band of Planetscope imagery.
Framing – Image Mosaicking and Clipping
It’s rare that a user’s area of interest (AOI) lines up precisely with the framing of a single image. A user will typically want to clip to a small area within an image or mosaic a number of scenes together and clip to their exact AOI boundaries for a larger area. These common tasks are being added to an upcoming version of Planet’s delivery API, and can be applied to bulk orders of imagery to ensure the user is only downloading the pixels they need with a single API call.
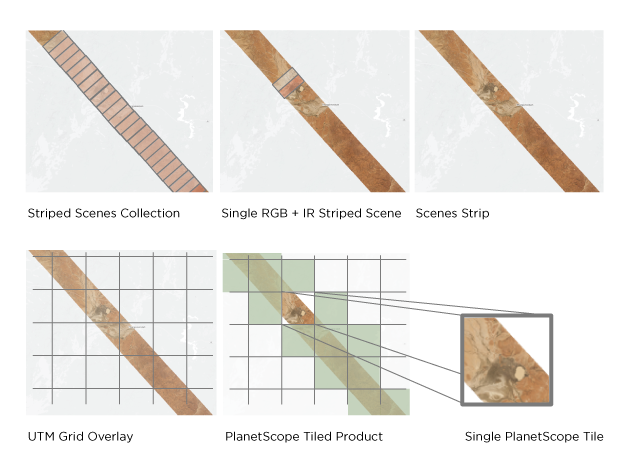
In our PlanetScope Ortho Tile product, imagery is collected as a series of overlapping framed scenes from a single satellite in a single pass. These scenes are subsequently orthorectified and an ortho tile is then generated from a collection of consecutive scenes.
Putting it All Together
The unifying vision for these components is to allow a user to generate ARDs on demand through our image delivery API, which can be delivered to a user’s cloud bucket and for further analysis as cloud optimized geotiffs. Imagine easily generating a growing season ARD cube clipped to an individual farmer’s field that includes daily PlanetScope imagery, weekly RapidEye imagery (including the red-edge band), and a Landsat image with SWIR every two weeks. If you can compile this data set in one easy step, your time is focused on using NDVI to assess general crop performance daily, nitrogen uptake every week with NDRE, and keeping an eye on water stress through evapotranspiration with Landsat’s thermal band, all together in a well-aligned data set.
If you’re interested in the topic of ARD and what it might look like in the future, I highly recommend you check out my colleague Chris Holmes recent two–part series on ARD. He presents a model for how the industry could standardize across sensors and platforms.