Slingshot Aerospace Maps Flooded Areas following Hurricane Harvey
When Hurricane Harvey made landfall in Houston earlier this month, Slingshot Aerospace, a Planet Application Developer Program partner, leapt into action. Working with Team Rubicon and the BAE Systems Geospatial eXploitation Products™ (GXP®), Slingshot was able to use its flood detection algorithms on up-to-date satellite imagery to determine the extent of the flooding and its severity. With this knowledge, they were able to highlight dry areas to assess search and rescue efforts to enable first responders and medical crews to safely reach those in need. Slingshot also used its algorithms to quickly identify flood relief and triage staging areas close to non-flooded roadways and optimal hospital routes. BAE Systems leveraged their cloud-hosted GXP solutions to provide Team Rubicon’s disaster response teams with the ability to visualize and analyze Planet imagery and Slingshot products.
Take a look at the mapping they’ve done in the storm’s aftermath:
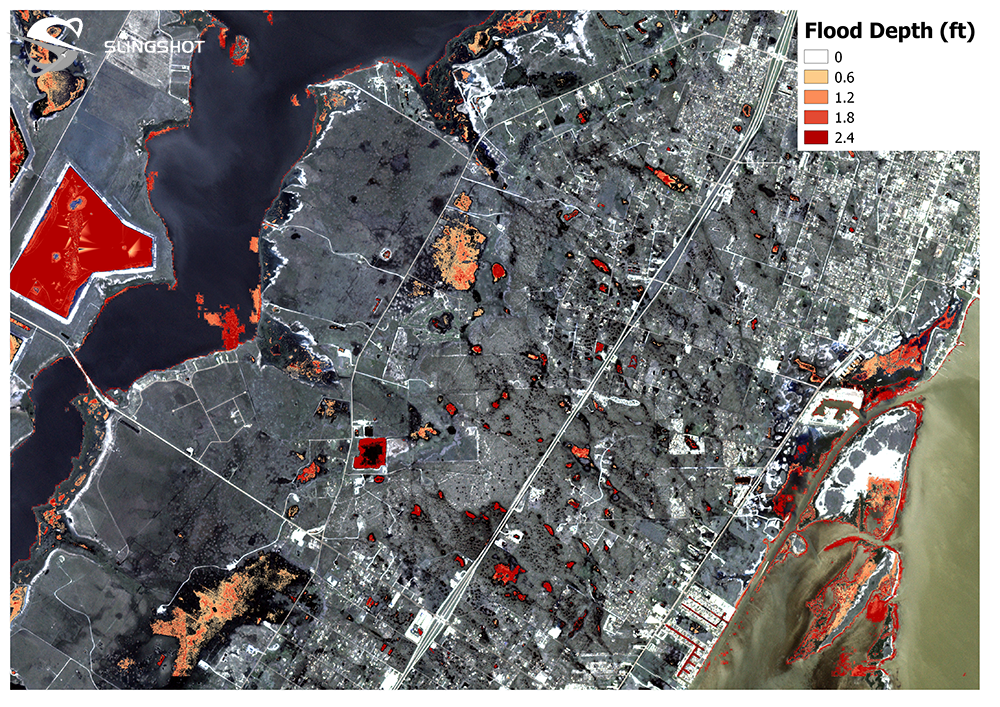
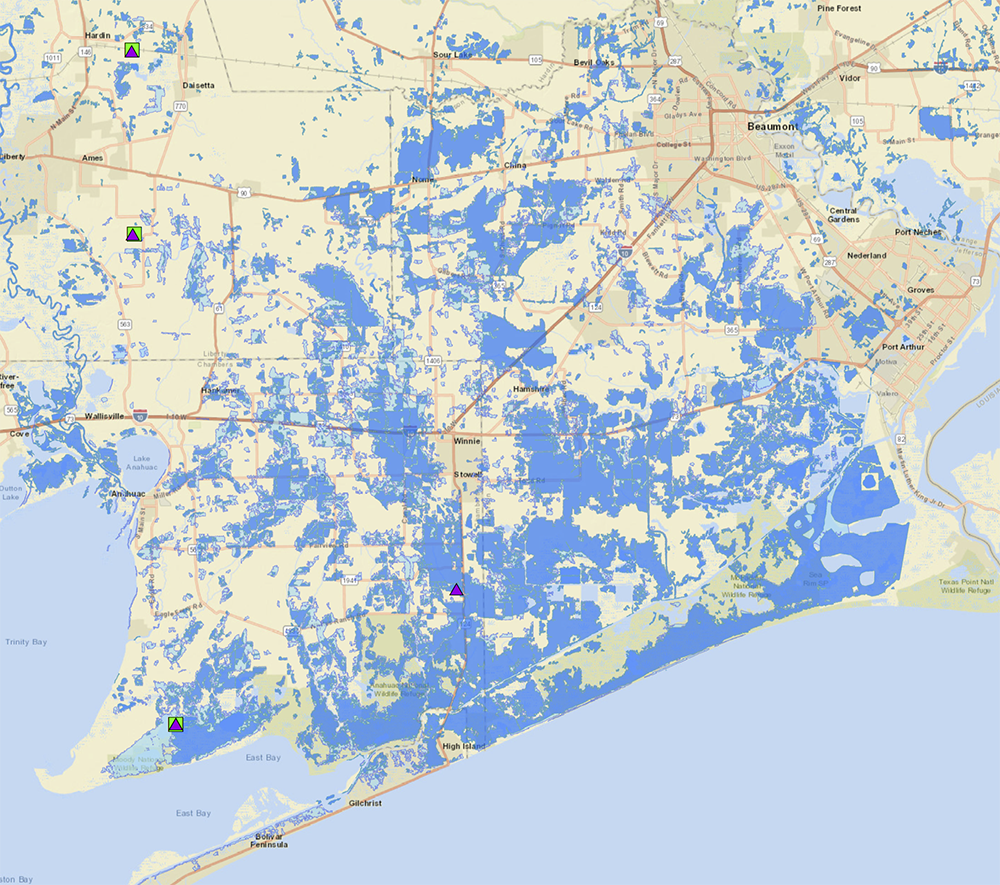
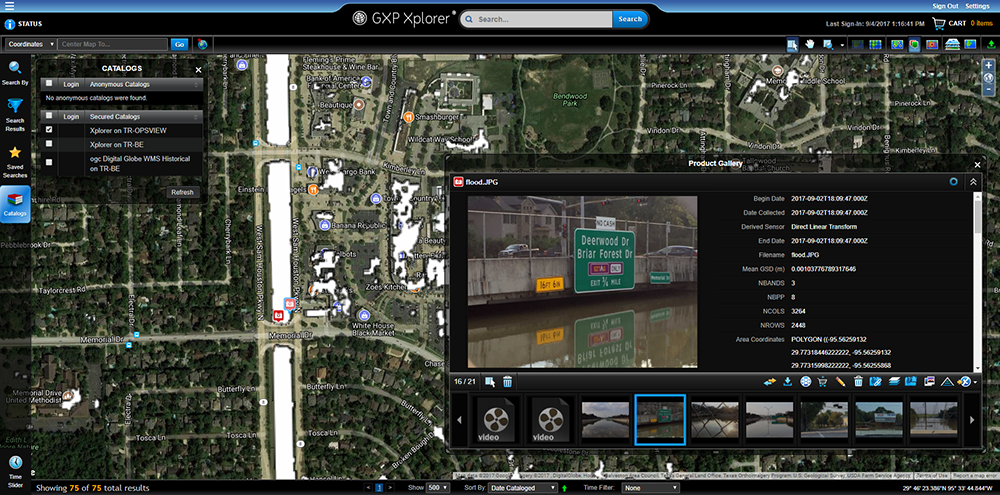
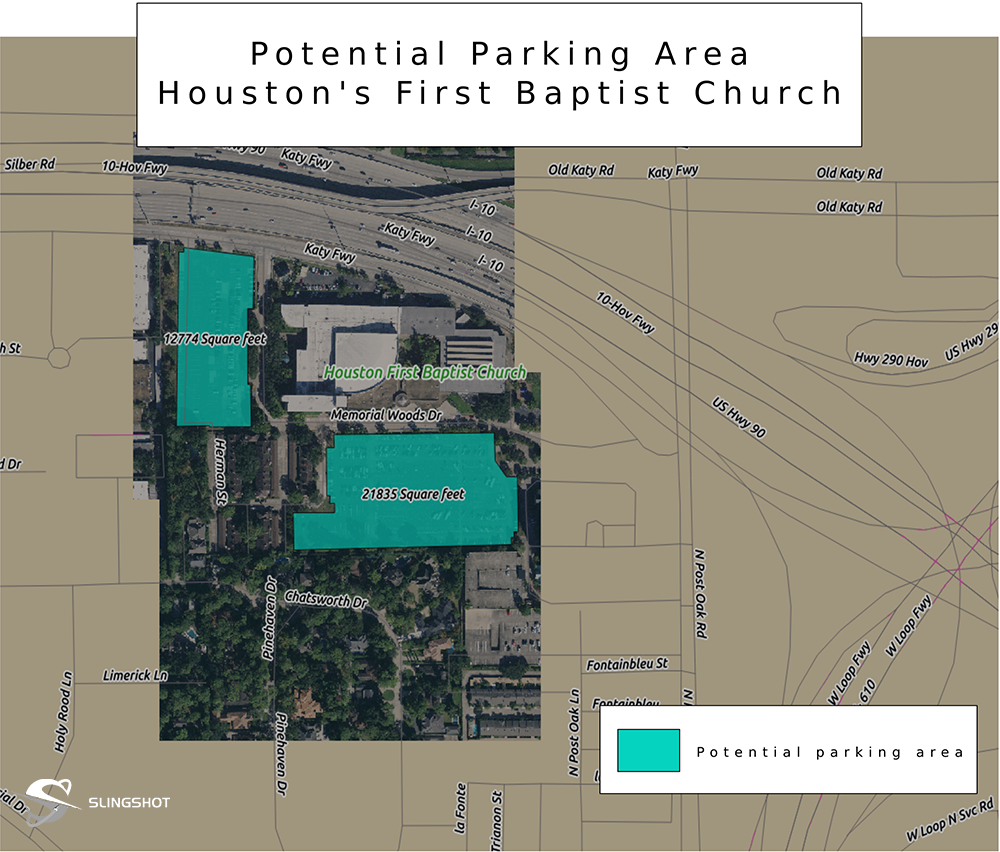
This is powerful example of how geospatial analytics and up-to-date imagery of anywhere can affect the speed and accuracy of disaster response and long-term recovery.